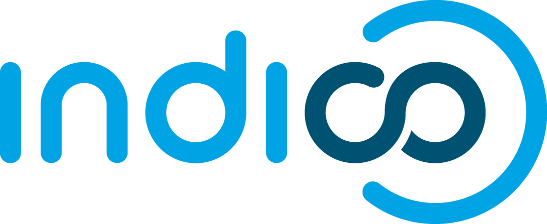
Please log in to access this page.
or change authentication provider
If you do not have an Indico account yet, you can create one here.
Your browser is out of date!
Update your browser to view this website correctly. Update my browser now